[Note: I don't have any personal race photos up yet; they should arrive in the next few days]
Finally back in Bloomington, after my standard sixteen-hour Megabus trip from Minnesota to Indiana, with a brief layover in Chicago to see The Bean. I had a successful run at Grandma's, almost six minutes faster than my previous marathon, which far exceeded my expectations going into it.
Both for any interested readers, and for my own personal use to see what I did leading up to and during race day, I've broken down the experience into segments:
1. Base Phase
I rebooted my training over winter break coming back from a brief running furlough, after feeling sapped from the Chicago Marathon. I began running around Christmas, starting with about 30 miles a week and building up to about 50 miles a week, but rarely going above that for some months. Now that I look back on it, this really isn't much of a base phase at all, at least according to the books I've read and runners I've talked to; but that was the way it worked out with graduate school and my other obligations.
Here are the miles per week, for eleven weeks, leading up to Grandma's:
Week 1: 38
Week 2: 57
Week 3: 43
Week 4: 55
Week 5: 45
Week 6: 48
Week 7: 70
Week 8: 31
Week 9: 80
Week 10: 29
Race Week: 28 (not including the marathon)
2. Workouts
The only workouts I do are 1) Long runs, typically 20-25 miles; and 2) Tempo runs, usually 12-16 miles at just above (i.e., slower than) threshold pace, which for me is around 5:40-5:50 a mile. That's it. I don't do any speedwork or interval training, which is a huge weakness for me; if I ever go under my lactate threshold too early, I crash hard. It has probably been about two years since I did a real interval workout, and I should probably get back to doing those sometime. The only thing is, I have never gotten injured or felt fatigued for days after doing a tempo run, whereas I would get stale and injured rather easily doing interval and speed workouts. In all likelihood I was doing them at a higher intensity than I should have when I was doing them.
3. TV Shows
The only TV show that I really watch right now is Breaking Bad, and the new season doesn't begin until July 15th. So, I don't have much to say about that right now.
4. Race Day
In the week leading up to the race, I was checking the weather reports compulsively. The weather for events like this can be fickle down to the hour, and the evening before the race I discussed race strategy with my dad. He said that in conditions like these, I would be wise to go out conservative and run the first couple of miles in the 6:00 range. It seemed like a sound plan, considering that I had done a small tune-up run the day before in what I assumed would be similar conditions, and the humidity got to me pretty quickly. In short, I expected to run about the same time I did at the Twin Cities marathon in 2010.
The morning of the race, the weather outside was cooler and drier than I expected, with a refreshing breeze coming in from Lake Superior. I boarded the bus and began the long drive to the start line, which really makes you realize how far you have to run to get back. I sat next to some guy who looked intense and pissed off and who I knew wouldn't talk to me and started pounding water.
At the starting line everything was warm and cheerful and sun-soaked and participants began lining up according to times posted next to the corrals. I talked to some old-timer who had done the race every single year, who told me about the good old days and how there used to only be ten port-a-johns, and how everyone would go to that ditch over yonder where the grassy slopes would turn slick and putrid. I said that sounded disgusting, and quickly walked over to my corral to escape.
Miles 1-6
At the start of the race, I decided to just go out on feel and take it from there. The first mile was quickly over, and at the mile marker my watch read: 5:42. I thought that I might be getting sucked out into a faster pace than I anticipated, but since I was feeling solid, I thought I would keep the same effort, if not back off a little bit, and then see what would happen. To my surprise, the mile splits got progressively faster: 5:39, 5:36, 5:37, 5:34, 5:33. I hit the 10k in about 34:54 and started to have flashbacks to Chicago last fall, where I went out at a similar pace and blew up just over halfway through the race. However, the weather seemed to be holding up, and actually getting better, instead of getting hotter and more humid. At this point my body was still feeling fine so I settled in and chose to maintain the same effort for the next few miles.
Miles 7-13
Things proceeded as expected, with a 5:34, 5:38, and 5:35 bringing me to mile 9. I saw my parents again at this point, both looking surprised that I had come through this checkpoint several minutes faster than planned. Although I knew it was fast, I didn't really care; at this point the weather had become decidedly drier than it was at the start of the race, the crowd was amping up the energy, and I was feeling bulletproof. It was also right about this time that I started feeling a twinge in my upper left quadricep.
Shit.
I had been having problems with this area for the past year, but for the last month things had settled down and it hadn't prevented my completing any workouts for the past few weeks. Still, it was a huge question mark going into the race, and with over sixteen miles to go, it could put me in the hurt locker and possibly force me to pull out of the race. I knew from experience that slowing down wouldn't help it so I continued at the same pace, hoping that it might go away.
5:28, 5:40, 5:37, 5:44. Although I was able to hold the same pace, the quadricep was getting noticeably worse, and began to affect my stride. I ignored it as best I could, trying instead to focus on the fact that I had come through the half in 1:13:40, which was close to a personal best and almost identical to what I had done at Chicago. I kept going, prepared to drop out at the next checkpoint if my leg continued to get worse.
Miles 14-20
The next couple of miles were quicker, with a 5:28 and 5:38 thanks to a gradual downhill during that section of the race. As I ran by the fifteen-mile marker, I began to notice that my quadricep was improving. The next pair of miles were 5:42, 5:42. At this point there was no mistaking it: The pain had completely disappeared, something that has never happened to me before. Sometimes I am able to run through mild injuries, but for them to recover during the course of a race, was new to me.
In any case, I didn't dwell on it. The next few miles flew by in 5:48, 5:38, and 5:55, and I knew that past the twenty-mile mark things were going to get ugly.
Miles 21-26.2
As we began our descent into the outskirts of Duluth, the crowds became larger and more frequent, clusters of bystanders serried together with all sorts of noisemakers and signs that sometimes had my name on them, although they were meant for someone else. At every aid station I doused myself with water and rubbed cool sponges over my face and shoulders, trying not to overheat. 5:57, 5:56. However, even though I was slowing way down, mentally I was still with it and able to focus. We had entered the residential neighborhoods, and the isolated bands of people had blended together into one long, continuous stream of spectators lining both sides of the road. I made every attempt to avoid the cracks in the street, sensing that if I made an uneven step, I might fall flat on my face.
5:55. Good, not slowing down too much. Still a few miles out, but from here I can see the promontory where the finish line is. At least, that's where I think it is. If that's not it, I'm screwed. Why are these morons trying to high-five me? Get the hell out of my way, junior. Just up and over this bridge. Do they really have to make them this steep? The clown who designed these bridges should be dragged into the street and shot. 6:03. I was hoping I wouldn't have any of those. I can still reel in a few of these people in front of me. This guy here is hurting bad; I would feel sorry for him, but at the same time it's such a rush to pass people when they are in a world of pain; almost like a little Christmas present each time it happens. 6:03 again. Oof. We've made another turn; things are getting weird. Where's the finish? Where am I? What's my favorite food? I can't remember. This downhill is steep as a mother, and I don't know if those muscles in my thighs serving as shock pads can take much more of this. Legs are burning, arms are going numb, lips are cracked and salty. One more mile to go here; just don't throw up and don't lose control of your bowels in front of the cameras and you're golden. 6:11. Whatever. There's the finish line; finally. Wave to the crowd; that's right, cheer me on, dammit! Almost there...and...done.
YES.
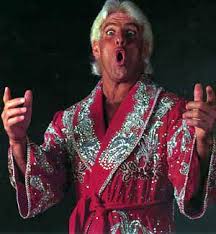 |
Artist's rendering of what I looked like at the finish |
29th overall, with a time of 2:30:23. I staggered around the finish area, elated and exhausted, starting to feeling the numbness creep into my muscles. For the next few days I had soreness throughout my entire body, but no hematuria, which is a W in my book.
More results can be found
here, as well as a summary page
here with my splits and a video showcasing my raw masculinity as I cross the finish line.
Next marathon will be in Milwaukee in October, and I'll be sure to give some running updates as the summer progresses.